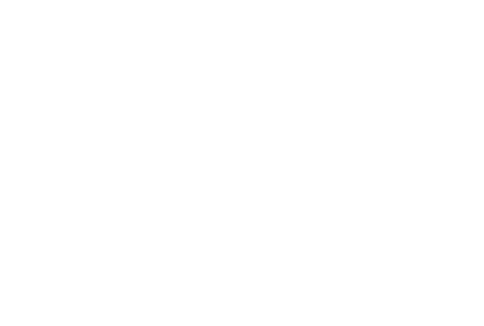
Project Details
Funding number: 03EE2032
Duration: 01.05.2021 – 30.04.2024
The ADWENTURE Project
Predictive maintenance is a key concept in operation and maintenance to reduce failures and unplanned down-times while improving equipment efficiency by the use of machine learning algorithms.
First, operational data is being analysed for anomalies within the time series. This information, however, is not yet sufficient to apply preventive maintenance actions. For this purpose additionally an interpretation of the anomaly which describes the type of probable error is required. Only with this information a specific maintenance activity can be performed to correct the detected issue.
The objective is to develop an automatic interpretation of detected anomalies by combining operational data with event and service report data. In addition to the application in turbine monitoring on the system level the project will also look into methods for anomaly detection in structural health and electrical component monitoring.
Furthermore, a round robin test for different anomaly detection software will be conducted based on a sample data set from the project.
Starting with an anomaly detection based on an autoencoder additional features will be integrated into the process. Different approaches to aggregate and interpret the reconstruction errors in regard of the criticality for the turbine will be tested. Transfer learning approaches will be applied to make use of the data from all turbines within a farm and to enable the transfer from trained models to turbines of the same type with an unknown behaviour and data history.
The methods will be tested on three wind farms. Detected anomalies will be analysed with pattern recognition approaches. Patterns that can be identified will then be linked to service actions documented in service reports. These links will additionally be assessed from an technical and analytical point of view.
As final outcome an algorithm will be developed that is able to classify anomalies automatically and to suggest suitable maintenance actions.
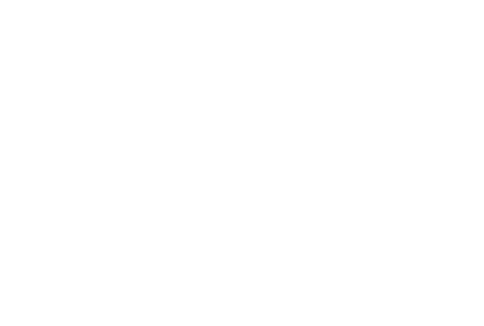